Introduction
Do you ever feel inundated with data when making a decision? So much so that you don’t know where to start. And even when you do start, it seems like you can never get a holistic view of what’s happening in your business.
You’re not alone. The average CEO is involved in 140 tasks a week, requiring many decisions. Fifty percent of their decisions were made in nine minutes or less. But there is hope. Decision intelligence is the new trending tool that can help you make sense of all your data, get a clear view of your business, and help support better decision-making.
Decision intelligence (DI) is an analytics field focusing on automated decision-making. It uses artificial intelligence (AI) techniques such as machine learning, natural language processing (NLP), and predictive modelling to automate data analysis and inform decisions. DI is very effective in fraud detection, customer churn prediction, and price optimisation.
This article will discuss what DI is, how it works, and some real-world applications. We will also provide tips on how to get started with Decision Intelligence in your organisation.
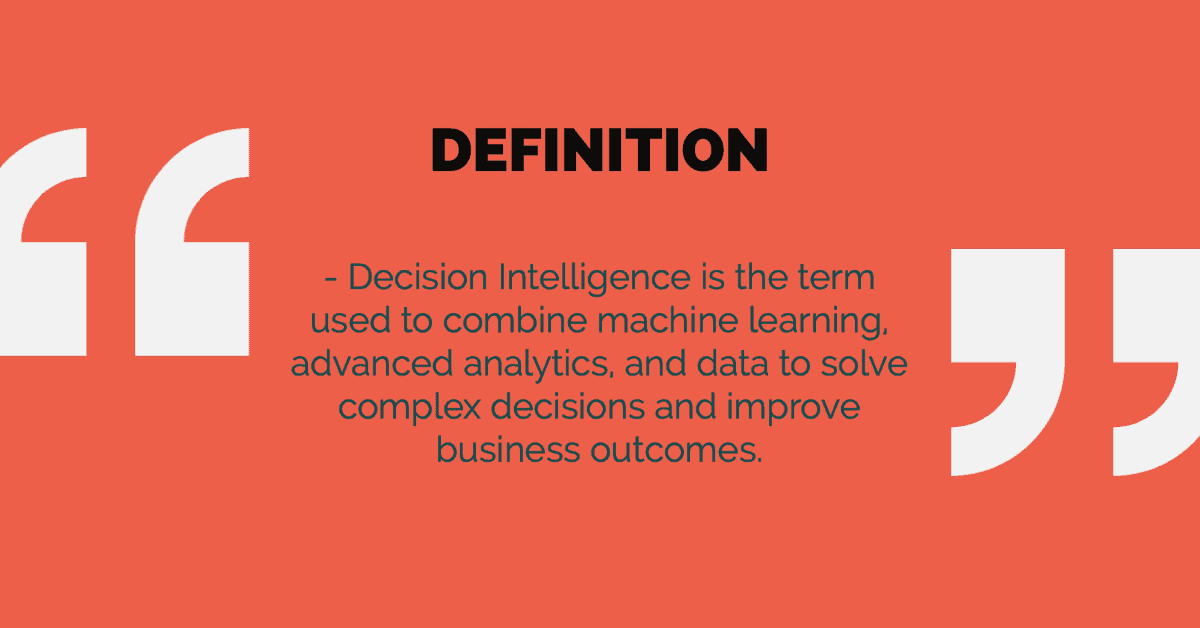
Contents:
-
What is Decision Intelligence?
-
Tell me the difference between Artificial Intelligence and Decision Intelligence
-
Why are algorithms as a service important now?
-
What are the benefits?
-
How does it work?
-
What are some common uses for Decision Intelligence?
-
What are the downsides?
-
What’s next for Decision Intelligence?
-
How do I start using DI in decision-making processes?
-
Conclusion
1. Terms frequently used in this post
2. References
PART I
1. What is Decision Intelligence?
Decision Intelligence, or DI for short, is the term given to an essential software category in which comprehensive decision support platforms use machine learning algorithms, data analytics, and decision modelling to solve complex decisions and improve business outcomes.
At the core of Decision Intelligence is the principle that all good decisions hinge on how actions lead to outcomes. Consequently, DI seeks to provide businesses with a holistic overview of their entire organisation. It allows them to identify opportunities, develop strategies, shape plans, launch projects, predict outcomes, and make decisions quickly.
Decision intelligence models enable companies to optimise their efforts by providing valuable insights into business operations at every level of business activity.
2. Tell me the difference between Artificial Intelligence and Decision Intelligence
Artificial Intelligence is the general term used to describe the area of data science capable of performing certain tasks automatically that traditionally only humans can do, such as self-driving cars.
Conversely, Decision Intelligence is the term that combines machine learning models and business analytics techniques to help improve business outcomes. Decision Intelligence doesn’t seek to replace human involvement; instead, it helps people make better choices with the assistance of Data Science. Examples include powering recommendation engines, language processing, or visual perception.
3. Why is Decision Intelligence important now?
Organisations need to be able to make sense of this deluge of information and use it to their advantage. Decision Intelligence can help them do this by providing a framework for making consistent data-driven decisions. In addition, generating actionable insights enables businesses to make the right decisions at the right time, leading to improved outcomes for the company and its customers.
In business, value is created by the outcome of its processes. Even the most straightforward procedures will consist of numerous decision points; see the diagram below.
The decision intelligence process can also provide flexibility. For example, in the case of black swan events like the COVID pandemic, many companies found the previous year’s data quickly became irrelevant as it no longer represented the new norm. Consequently, any machine-learning predictions built using this information were no longer helpful.
DI has undertaken all the complex analysis, leading to faster decisions and enabling businesses to invest their internal resources elsewhere, resulting in a quicker return on investment and greater profits.
Decision Intelligence can help solve these problems by facilitating complex problem-solving capabilities.
4. What are the benefits?
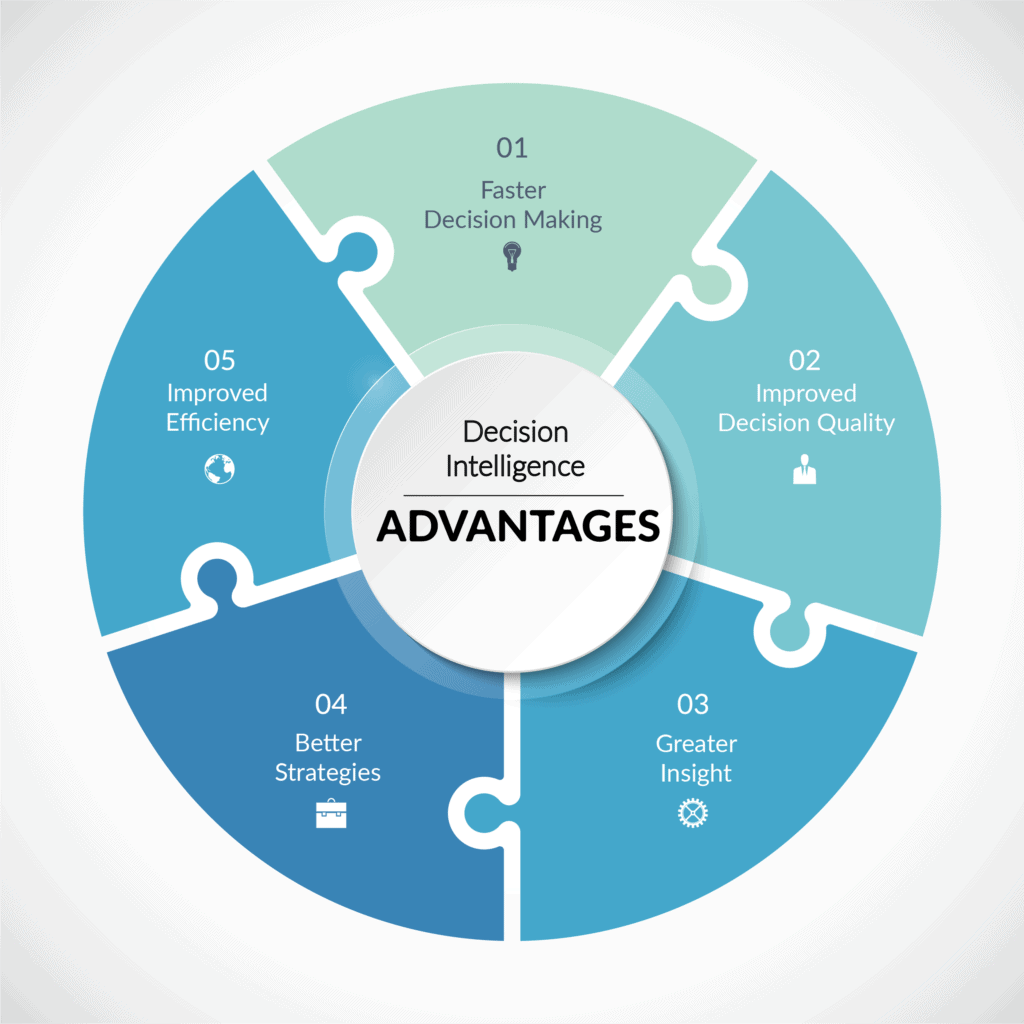
Faster Decision Making
Decision intelligence can help you make decisions faster by providing you with the insights you need to make an informed decision.
Improved Decision Quality
Decision intelligence can also improve the quality of your decisions by helping you to identify and assess all relevant information.
Greater Insight
Decision intelligence provides greater insight into your business operations and a better understanding of what is happening and why. It can automate tasks traditionally executed by technical teams to transform data dashboards.
Better Strategies
Decision intelligence can help you develop better strategies by providing insights into your business that you may not have otherwise considered.
Improved Efficiency
Decision intelligence can improve the efficiency of your decision-making process by helping you to focus on the most relevant information.
5. How does it work?
Decision intelligence uses principles from social science, decision theory, and managerial science.
Its purpose is to provide a set of best practices for organisational decision-making and to develop methods for applying machine learning at scale.
Data science techniques help decision intelligence understand business trends and scenarios. To do this, the system needs to collect data from all parts of the company. The more data that is collected, the better the algorithm will understand how your company works.
Decision intelligence solutions then use machine learning and natural language processing to understand the data better. This is done by looking at how actions lead to outcomes.
Decision Intelligence Framework
Decision-making made easy. Combining 5 data science advanced disciplines
-
- Deep learning
- Data dashboards and visualisations
- Future predictive analytics
- Machine learning decision models
- Complex ai models
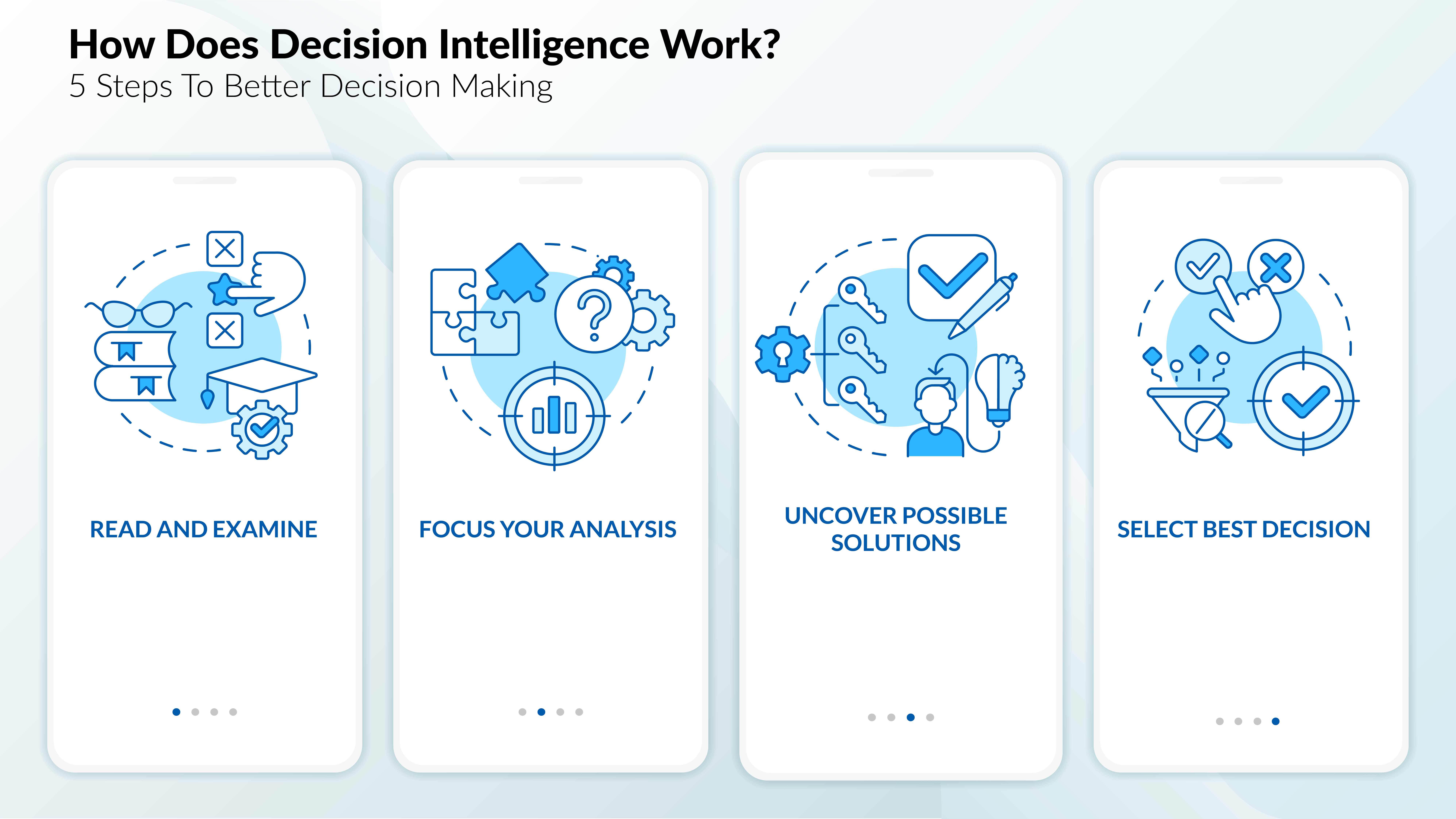
Data Intelligence solutions are comprised of three main components.
Data
Data is paramount for any technology or software that plans to be precise. Data Science models process the data and determine the correct insights to support business decision-making. The more information fed into the models, the more accurate their predictions become.
DI helps dismantle the barriers that impede communication between various data systems. Consequently, this makes it easier for different departments within a company to collaborate and be outcome focused.
Machine Learning Algorithms
Machine learning technology identifies patterns, correlations, and future trends in the data. In turn, businesses have more capacity to focus on their core goals while the DI solution takes care of analysing all the data and generating insights. Decision-making processes are accelerated and improved.
You can predict results in real-time by using machine learning to analyse and model business decisions. Business leaders should note that Decision Intelligence doesn’t seek to replace human involvement; instead, it helps people make better choices with the assistance of Data Science.
Algorithms as a Service: The Future of Computing
Algorithms as a Service represent a new category of software that allows you to use pre-built algorithms without developing and maintaining them. This article will explore algorithms as a service and how they will change how businesses operate.
Infrastructure
Infrastructure includes specialised hardware, software, and cloud services that support data ingestion processes and algorithms. Many DI providers use third-party Cloud solutions because they are easy to set up and don’t require businesses to worry about supplying or maintaining their hardware.
PART II
1. What are some common uses for Decision Intelligence?
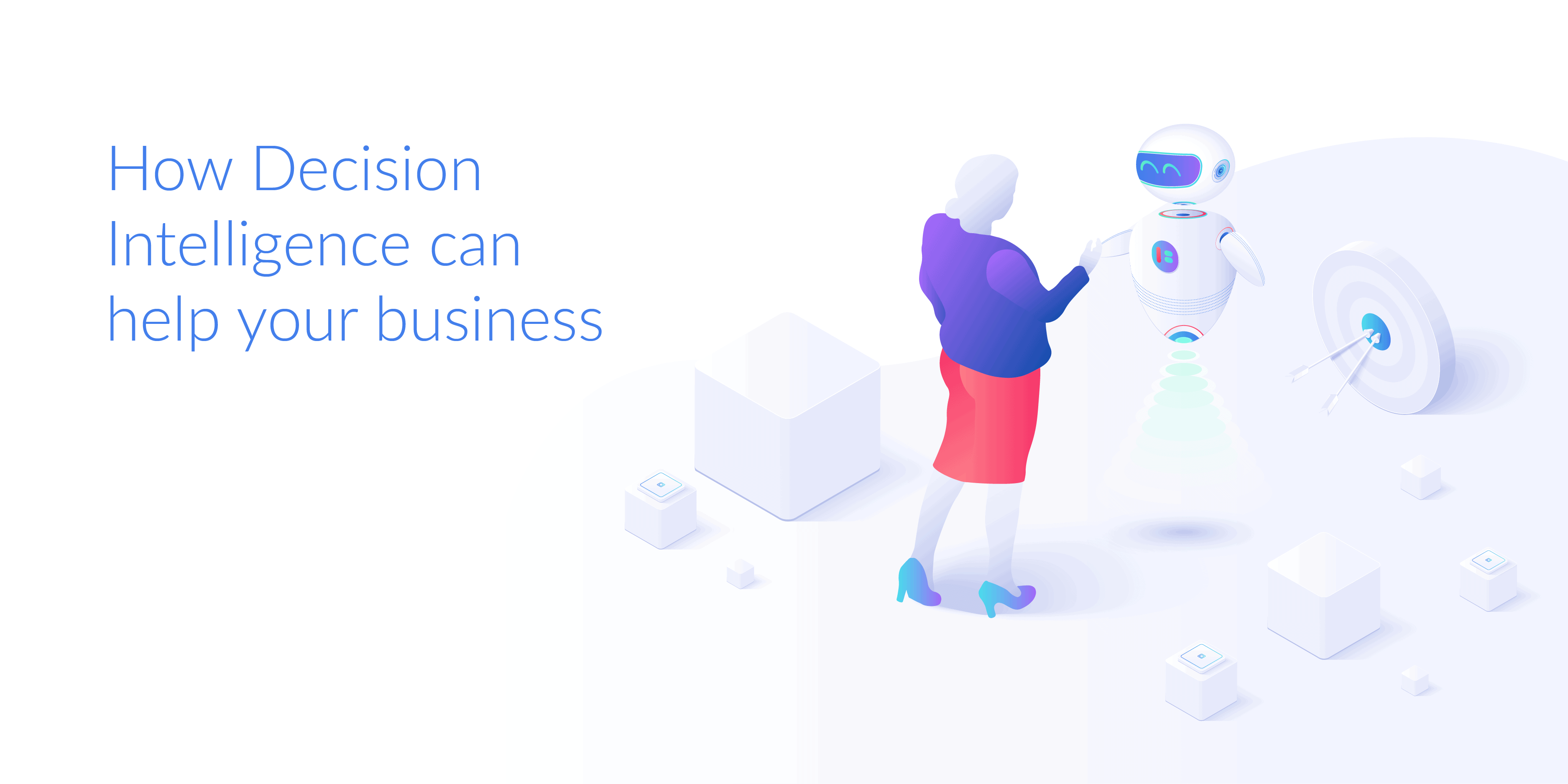
Decision Intelligence can help organisations in any industry, including marketing, demand management, supply chain, logistics, etc.
The following represents a practical application of DI taken from the comprehensive IDC report – It’s time to improve your organisation with decision intelligence.
Example 1 – Aksigorta
Aksigorta, an insurance company, was lagging behind its competitors because it struggled to use an industry-standard auto policy pricing model. It decided to tune decision models and developed a new AI-based pricing model. The company improved its understanding of each customer’s risk level with this new approach. This allowed Aksigorta to make more competitive policy pricing decisions, and as a result, the company’s share in the Turkish passenger car market increased by 55%. There was also a 237% increase in light commercial vehicles, and the overall margin went from 15% to 23%.
Example 2 -Carhartt
Carhartt analyse data to build a tool to predict demand at a postal code level. This includes consumer behaviour, customer demographics, past sales, supply chain, employment numbers, climate, and socioeconomic conditions. With this new information readily available, the company had a much better understanding of which locations would present the most revenue opportunity. The decision intelligence tool proved itself almost immediately – in 2019, after opening three new stores using the predictions generated by the device; December sales for those locations exceeded original projections by 200%.
Example 3 – Molslinjen
Molslinjen, a Danish ferry operator, increased the accuracy of its demand forecasts by using AI-based forecasting instead of relying on historical statistics and management experience. The new system is 70% more accurate, resulting in over 4500 additional tickets sold annually from business customers alone.
Example 4 -ASOS
ASOS, a U.K.based online fashion retailer, decided that they needed to increase the productivity of those working in their distribution centre. They did so by considering several key metrics and then using decision intelligence in real-time, which allowed them to see an increase of 5% in picks per person per minute.
Example 5 -ENGIE
After modernising its data management, analytics, and AI approach, French utility company ENGIE began leveraging decision intelligence across its business. Today it has developed around 300 AI-powered use cases, including:
- A system that monitors and regulates the noise made by wind turbines
- Predictive maintenance systems save an estimated €800,000 annually by monitoring 10,000 pieces of equipment.
- Predict how much solar power will be produced.
2. What are the downsides?
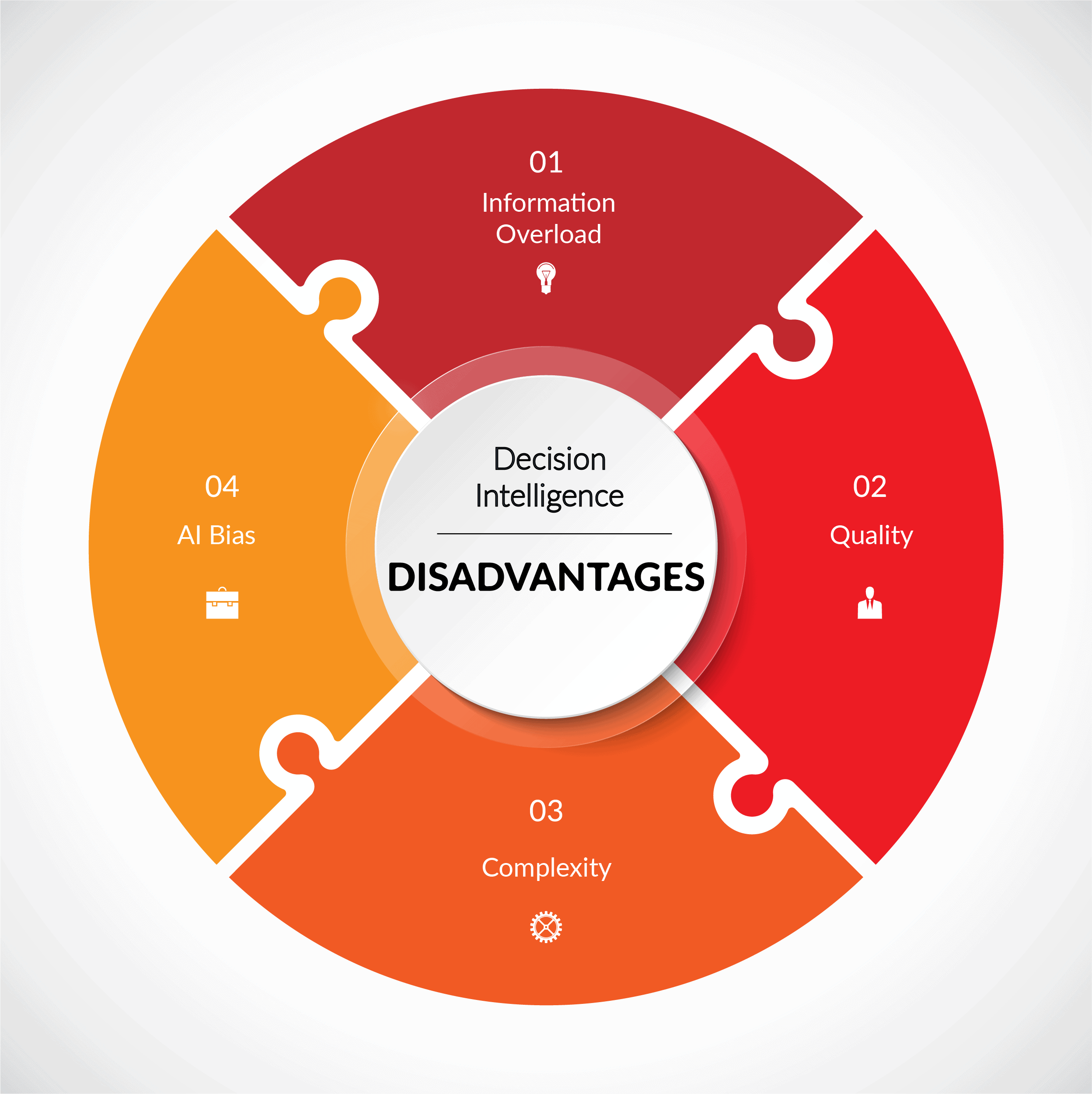
Information Overload
Making decisions is hard when you are faced with too much data. It is hard to see the woods for the trees when you have volumes of information to consider.
Quality
A common obstacle to decision intelligence is data quality. With an excess of information available, it can be tough to know which data is accurate and which isn’t.
Complexity
Because decision intelligence can be complex, it may be challenging to understand and use.
AI Bias
There are two types of data science-related bias: algorithmic or “data” bias and societal bias. The former is where algorithms learn from biased data; the latter occurs when humans’ assumptions and norms influence our way of thinking.
Although decision intelligence comes with some challenges, it is still a valuable tool that can help you make decisions more effectively for your business. If you want to improve how you go about making decisions, think about implementing decision intelligence into your process.
3. What’s next for Decision Intelligence?
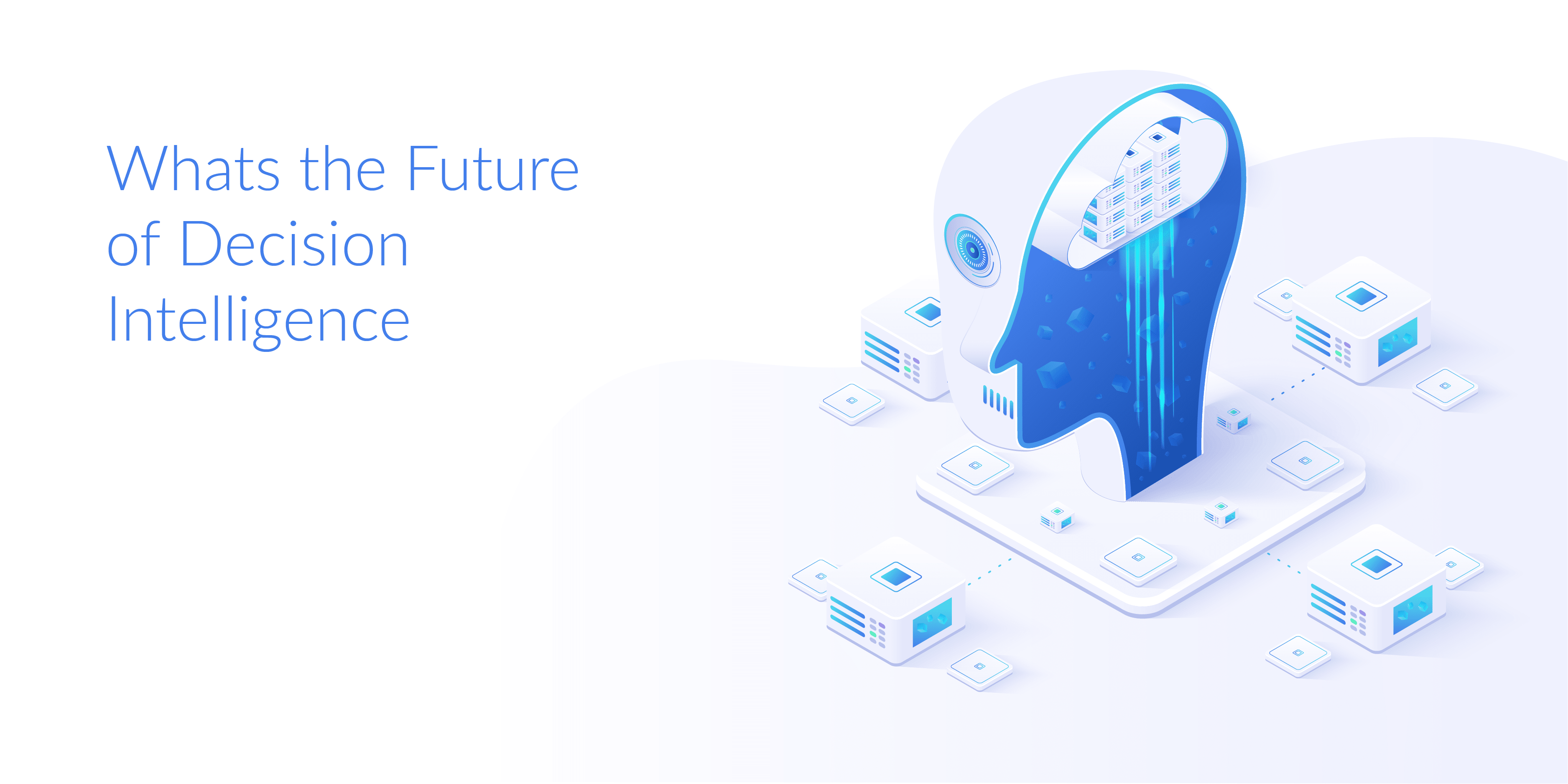
Strategic technology decisions are more critical than ever, and according to Gartner, decision intelligence is one of this year’s top trends. Gartner predicts that by 2023, more than a third of large organisations will be using some form of decision intelligence practices. Organisations need to make decisions faster than ever before — and on a scale not yet seen.
Decision Intelligence will be how many enterprises adopt AI, helping provide an automated way to make decisions, which will help companies keep up and outpace their competitors.
Data has been siloed in the past, making it difficult for businesses to consider all the information when making decisions. However, with DI, anyone in an organisation can access to business intelligence, resulting in more informed and consistent decision-making.
4. How do I start using DI in decision-making processes?
To start, identify a complex business process and collect as much supporting data as possible.
Next, identify and train an appropriate machine learning algorithm, validating along the way the results generated are valid. Finally, embed the new insight into the decision-making process to help determine the proper outcome(s).
You can ease the process by beginning with a well-identified, low-risk project encompassing a large sample set. Adding more factors to an existing process can enhance accuracy and reduce the system’s complexity.
After initial use cases, gradually scale decision intelligence company-wide.
5. Conclusion
The impact of digital disruption continues to magnify the complexity of both business problems and decision-making processes.
Companies create value from their business processes, composed of many different decision waypoints. By learning how various elements influence the outcome of a decision, businesses can enhance and improve their decision-making process. Companies that immediately implement new technologies, such as Advanced Decision Intelligence, will experience advantages from extensive innovation and remain ahead of other companies in their field.
Decision Intelligence is fast becoming a popular approach that helps businesses address specific problems using data science techniques. Rather than AI technologies triggering wide-scale digital transformations, Decision Intelligence provides the commercial application with which companies can scale their Data Science capabilities across the organisation.
With this tool, those in charge can make decisions more quickly and efficiently. Therefore, the company can improve its decision-making process leading to better business outcomes.
What impact do you think Decision Intelligence will have on your business?
Please comment below to share your thoughts on this blog post.
PART III
1. Terms frequently used in the post
What are algorithms?
An algorithm can be defined as instructions for solving a problem or accomplishing a task. There are various types of algorithms, which can be broken down into different categories.
Artificial intelligence algorithms are a subset of algorithms that have been specifically designed to simulate or perform human-like tasks.
Types of algorithms
Supervised algorithms are named as such, as they are given data that has been labelled upon which to “learn”. The algorithms iteratively make predictions and then are adjusted to the correct answer using this training data. This means the algorithm becomes optimised to know the right solution for each data item it processes.
Supervised learning is like learning from a textbook. The algorithm learns from training data and then applies that knowledge to new data. Supervised learning models are typically more accurate than unsupervised ones but require human intervention to label the data.
An example of a supervised algorithm would be a model that can predict how long your car journey will be based on the time of day. But first, this model needs training to know that rainy weather will likely extend the driving time.
Unsupervised algorithms are given unlabelled data from which to learn. The algorithm works independently to discover hidden patterns or groupings within the data.
Unsupervised learning is like learning from experience, and they make an ideal solution when exploring data or making recommendations.
Such algorithms have many advantages, but there are some risks. To avoid biased outcomes, AI developers must rely on human verification to decide what is reasonable. Transparency into why specific associations have been made is crucial as unsupervised algorithms can provide little insight into how they reached conclusions about the data.
An example of an unsupervised algorithm would be a model that can identify the behaviours of online shoppers who often purchase groups of products simultaneously. However, to show whether it makes sense to combine paper with an order of pens, pencils, and erasers, you need to validate that it makes sense.
Semi-supervised algorithms use data that is only partially labelled. In this scenario, the algorithm uses labelled data to learn and fill in the gaps of the rest of the unlabelled data. Using a semi-supervised algorithm is that it can make informed assumptions based on the labelled data.
This algorithm is widely used in image recognition, where much of the data is unlabelled. The algorithm can learn how to classify objects by referencing pictures that have been correctly labelled and categorised. It can also learn to recognise objects in photos that have not been tagged.
The cost to label data appropriately is often high due to the expertise and time required. So, semi-supervised or unsupervised algorithms can be a more appealing alternative without fully labelled data.
What is machine learning?
Machine learning is a term used to describe algorithms that learn from data.
They apply this learning to make predictions about new data. The accuracy of these predictions automatically improves over time as the algorithm processes more data.
Types of machine learning:
-
-
- Linear regression
- Logistic regression
- Decision tree
- Random forest
- Neural networks
-
ML Managed Services
The vendor manages all aspects of the machine learning models for you, from training to deployment.
Pre-trained Models
These machine-learning models have already been trained on specific data sets and are ready to be used.
These machine learning models can be customised to your specific data set and needs.
Customisable models may be the best option if you have the time and expertise. Pre-trained models may be better if you need a quick solution ready to use. And if you want someone else to take care of everything for you, managed services are the way to go.
2. References
Decision intelligence. (2022, October 9). In Wikipedia.
The Basics of Decision Intelligence. (2021, Dec 26). In AnalyticSteps.
What is “Decision Intelligence” and How Do I Get It?. (2022, June 15). In IT/IQ Tech Recruiters.
How to make choosing easier. (2011). In Ted.
IDC Report: Level up your organization with Decision Intelligence. (2021, October). In Peak AI.
Task: Identify Decision Points. (2017). In ESROCOS Consortium.
Gartner Glossary – Decision Intelligence. (2022). In Gartner.
What is decision intelligence, and where has it come from? (2022, January 5). In BCS, The Chartered Institute for IT.
Decision Intelligence: Definition and Examples. (2022, May 3). In CIOInsight.
How Much Data is Created Every Day in 2022? (2022, August 18). In WP Dev Shed.
The Basics of Decision Intelligence. (2021, December 26). In AnalyticSteps.
Bias in AI and Machine Learning: Sources and Solutions. (Unknown). In Lexalytics.